
Building on our previous post about scalable data storage, the next essential step for businesses is data transformation—ensuring data is "insight-ready" for effective decision-making. In this post, we’ll explore the role of data transformation in unlocking valuable insights, using FreshBytes as an example to highlight its benefits and impact.
The limitations of raw data for generating insights
Data often starts life off in raw, unstructured formats that may be incomplete, inconsistent, or dispersed across various systems. Without data transformation, companies face challenges in integrating sources, identifying patterns and drawing reliable conclusions.
FreshBytes needed a comprehensive view of the customer journey to understand issues like cart abandonment, supply chain inefficiencies and customer churn. However, fragmented data from website interactions, purchase history, inventory and loyalty programmes undermined confidence in their insights. This disjointed data limited their ability to forecast inventory and revenue, optimise store performance, and improve customer retention. Additionally, challenges in data quality, governance and collection further prevented FreshBytes from gaining actionable insights to drive targeted actions.
The need for reliable insight ready data
Every organisation, regardless of size, location or industry, needs clean, consistent, and insights-ready data to guide decision-making
Consolidating customer, sales and relevant data into a unified dataset, would enable a company like FreshBytes to gain a clearer picture of customer behaviour. This would allow it to identify the factors driving cart abandonment and take data-informed steps to improve satisfaction, retention and conversion rates.
Leveraging Data Transformation for Business Value
Data transformation is not a one-time task; it demands an ongoing, robust solution capable of handling complex datasets at scale.
Example: FreshBytes Lakehouse for insights
Data Integration
The lakehouse solution would unify data from multiple sources, including daily customer transaction data (500 GB compressed), customer support logs (500 GB daily), and customer records (500 GB daily). Additionally, three years of historical data (approximately 6 PB) could be included to enable a comprehensive analysis of customer behaviour and trends over time.
Data Processing
Using Apache Spark on the lakehouse architecture, raw data would be processed and transformed into analysis-ready datasets. This would involve performing complex joins and aggregations across the entire dataset to eliminate data silos and resolve the processing limitations that previously hindered complete analysis.
Advanced Analytics
Transformed data enables advanced data analytics, supporting predictive insights like inventory demand forecasting, customer behaviour prediction and forecasting resource utilisation. In addition, it offers operational intelligence which would include, real-time monitoring, automated anomaly detection, and dynamic resource allocation—driving proactive decisions and operational efficiency at FreshBytes.
Real-Time Insights
Streaming analytics would enable real-time monitoring of customer behaviour, allowing for immediate actions and adjustments. Automated alerts would notify teams of data inconsistencies, ensuring timely issue resolution and maintaining data accuracy and reliability.
The success behind lakehouse-powered insights
By consolidating real-time and historical data in a single platform, lakehouse solutions can help organisations make faster, insight-driven decisions, optimise operational costs, and foster a more data-centric culture across teams.
To illustrate, let’s take a look at how FreshBytes could benefit from adopting lakehouse-powered insights:
Customer Journey Analysis
By linking online behaviour and interaction data with transaction history, FreshBytes could trace the complete customer journey—from product browsing to cart addition to final checkout. This would help identify specific touchpoints where customers were dropping off, such as during the payment stage or after viewing shipping costs.
Segmentation and Personalisation
Leveraging customer segmentation models, FreshBytes could have grouped customers based on behaviour, purchase history, or demographics. They might have discovered that certain segments—like new visitors or mobile users—were more prone to abandon their carts. This would allow for targeted interventions, such as personalised recommendations or tailored discount offers.
Performance Improvements
By leveraging real-time and historical data, FreshBytes could dramatically reduce the time needed for analysis. What once took weeks might now be completed in hours, enabling faster, more responsive decision-making. With real-time monitoring, they could also identify customer issues more quickly, reducing response times from days to minutes.
Cost Efficiency
Through efficient data organisation and query optimisation, FreshBytes could realise significant cost savings, even when dealing with large datasets. For example, compute costs could potentially decrease by 40%, enabling more cost-effective data processing without sacrificing performance.
Business Impact
By identifying and removing duplicate customer records, FreshBytes could reduce marketing costs by approximately 15%, streamlining campaigns and targeting. Additionally, personalised recommendations—driven by segmentation insights—could increase average order value, driving higher revenue per customer. Real-time monitoring could also help the support team proactively assist customers, potentially boosting customer satisfaction scores.
Data-Driven Culture
As FreshBytes continues to leverage lakehouse-powered insights, it could foster a more data-driven culture throughout the organisation. With better access to accurate, real-time data, departments might become more inclined to base their decisions on evidence and insights. This could lead to a 300% increase in data queries across the company, as analytics become more deeply integrated into everyday operations.
From Data to Intelligence
The FreshBytes example illustrates the transformative impact of data transformation within a lakehouse architecture. By converting raw, fragmented data into unified, clean, and insight-ready formats organisations can overcome immediate data challenges and build a strong foundation for growth and innovation. If your organisation aims to turn raw data into actionable intelligence, HorizonX can guide you every step of the way. Contact us today to begin your journey to data excellence.
In our next post, we’ll explore the powerful applications of AI and Machine Learning (ML) in advanced analytics.
Subscribe for insights
Building on our previous post about scalable data storage, the next essential step for businesses is data transformation—ensuring data is "insight-ready" for effective decision-making. In this post, we’ll explore the role of data transformation in unlocking valuable insights, using FreshBytes as an example to highlight its benefits and impact.
The limitations of raw data for generating insights
Data often starts life off in raw, unstructured formats that may be incomplete, inconsistent, or dispersed across various systems. Without data transformation, companies face challenges in integrating sources, identifying patterns and drawing reliable conclusions.
FreshBytes needed a comprehensive view of the customer journey to understand issues like cart abandonment, supply chain inefficiencies and customer churn. However, fragmented data from website interactions, purchase history, inventory and loyalty programmes undermined confidence in their insights. This disjointed data limited their ability to forecast inventory and revenue, optimise store performance, and improve customer retention. Additionally, challenges in data quality, governance and collection further prevented FreshBytes from gaining actionable insights to drive targeted actions.
The need for reliable insight ready data
Every organisation, regardless of size, location or industry, needs clean, consistent, and insights-ready data to guide decision-making
Consolidating customer, sales and relevant data into a unified dataset, would enable a company like FreshBytes to gain a clearer picture of customer behaviour. This would allow it to identify the factors driving cart abandonment and take data-informed steps to improve satisfaction, retention and conversion rates.
Leveraging Data Transformation for Business Value
Data transformation is not a one-time task; it demands an ongoing, robust solution capable of handling complex datasets at scale.
Example: FreshBytes Lakehouse for insights
Data Integration
The lakehouse solution would unify data from multiple sources, including daily customer transaction data (500 GB compressed), customer support logs (500 GB daily), and customer records (500 GB daily). Additionally, three years of historical data (approximately 6 PB) could be included to enable a comprehensive analysis of customer behaviour and trends over time.
Data Processing
Using Apache Spark on the lakehouse architecture, raw data would be processed and transformed into analysis-ready datasets. This would involve performing complex joins and aggregations across the entire dataset to eliminate data silos and resolve the processing limitations that previously hindered complete analysis.
Advanced Analytics
Transformed data enables advanced data analytics, supporting predictive insights like inventory demand forecasting, customer behaviour prediction and forecasting resource utilisation. In addition, it offers operational intelligence which would include, real-time monitoring, automated anomaly detection, and dynamic resource allocation—driving proactive decisions and operational efficiency at FreshBytes.
Real-Time Insights
Streaming analytics would enable real-time monitoring of customer behaviour, allowing for immediate actions and adjustments. Automated alerts would notify teams of data inconsistencies, ensuring timely issue resolution and maintaining data accuracy and reliability.
The success behind lakehouse-powered insights
By consolidating real-time and historical data in a single platform, lakehouse solutions can help organisations make faster, insight-driven decisions, optimise operational costs, and foster a more data-centric culture across teams.
To illustrate, let’s take a look at how FreshBytes could benefit from adopting lakehouse-powered insights:
Customer Journey Analysis
By linking online behaviour and interaction data with transaction history, FreshBytes could trace the complete customer journey—from product browsing to cart addition to final checkout. This would help identify specific touchpoints where customers were dropping off, such as during the payment stage or after viewing shipping costs.
Segmentation and Personalisation
Leveraging customer segmentation models, FreshBytes could have grouped customers based on behaviour, purchase history, or demographics. They might have discovered that certain segments—like new visitors or mobile users—were more prone to abandon their carts. This would allow for targeted interventions, such as personalised recommendations or tailored discount offers.
Performance Improvements
By leveraging real-time and historical data, FreshBytes could dramatically reduce the time needed for analysis. What once took weeks might now be completed in hours, enabling faster, more responsive decision-making. With real-time monitoring, they could also identify customer issues more quickly, reducing response times from days to minutes.
Cost Efficiency
Through efficient data organisation and query optimisation, FreshBytes could realise significant cost savings, even when dealing with large datasets. For example, compute costs could potentially decrease by 40%, enabling more cost-effective data processing without sacrificing performance.
Business Impact
By identifying and removing duplicate customer records, FreshBytes could reduce marketing costs by approximately 15%, streamlining campaigns and targeting. Additionally, personalised recommendations—driven by segmentation insights—could increase average order value, driving higher revenue per customer. Real-time monitoring could also help the support team proactively assist customers, potentially boosting customer satisfaction scores.
Data-Driven Culture
As FreshBytes continues to leverage lakehouse-powered insights, it could foster a more data-driven culture throughout the organisation. With better access to accurate, real-time data, departments might become more inclined to base their decisions on evidence and insights. This could lead to a 300% increase in data queries across the company, as analytics become more deeply integrated into everyday operations.
From Data to Intelligence
The FreshBytes example illustrates the transformative impact of data transformation within a lakehouse architecture. By converting raw, fragmented data into unified, clean, and insight-ready formats organisations can overcome immediate data challenges and build a strong foundation for growth and innovation. If your organisation aims to turn raw data into actionable intelligence, HorizonX can guide you every step of the way. Contact us today to begin your journey to data excellence.
In our next post, we’ll explore the powerful applications of AI and Machine Learning (ML) in advanced analytics.
Building on our previous post about scalable data storage, the next essential step for businesses is data transformation—ensuring data is "insight-ready" for effective decision-making. In this post, we’ll explore the role of data transformation in unlocking valuable insights, using FreshBytes as an example to highlight its benefits and impact.
The limitations of raw data for generating insights
Data often starts life off in raw, unstructured formats that may be incomplete, inconsistent, or dispersed across various systems. Without data transformation, companies face challenges in integrating sources, identifying patterns and drawing reliable conclusions.
FreshBytes needed a comprehensive view of the customer journey to understand issues like cart abandonment, supply chain inefficiencies and customer churn. However, fragmented data from website interactions, purchase history, inventory and loyalty programmes undermined confidence in their insights. This disjointed data limited their ability to forecast inventory and revenue, optimise store performance, and improve customer retention. Additionally, challenges in data quality, governance and collection further prevented FreshBytes from gaining actionable insights to drive targeted actions.
The need for reliable insight ready data
Every organisation, regardless of size, location or industry, needs clean, consistent, and insights-ready data to guide decision-making
Consolidating customer, sales and relevant data into a unified dataset, would enable a company like FreshBytes to gain a clearer picture of customer behaviour. This would allow it to identify the factors driving cart abandonment and take data-informed steps to improve satisfaction, retention and conversion rates.
Leveraging Data Transformation for Business Value
Data transformation is not a one-time task; it demands an ongoing, robust solution capable of handling complex datasets at scale.
Example: FreshBytes Lakehouse for insights
Data Integration
The lakehouse solution would unify data from multiple sources, including daily customer transaction data (500 GB compressed), customer support logs (500 GB daily), and customer records (500 GB daily). Additionally, three years of historical data (approximately 6 PB) could be included to enable a comprehensive analysis of customer behaviour and trends over time.
Data Processing
Using Apache Spark on the lakehouse architecture, raw data would be processed and transformed into analysis-ready datasets. This would involve performing complex joins and aggregations across the entire dataset to eliminate data silos and resolve the processing limitations that previously hindered complete analysis.
Advanced Analytics
Transformed data enables advanced data analytics, supporting predictive insights like inventory demand forecasting, customer behaviour prediction and forecasting resource utilisation. In addition, it offers operational intelligence which would include, real-time monitoring, automated anomaly detection, and dynamic resource allocation—driving proactive decisions and operational efficiency at FreshBytes.
Real-Time Insights
Streaming analytics would enable real-time monitoring of customer behaviour, allowing for immediate actions and adjustments. Automated alerts would notify teams of data inconsistencies, ensuring timely issue resolution and maintaining data accuracy and reliability.
The success behind lakehouse-powered insights
By consolidating real-time and historical data in a single platform, lakehouse solutions can help organisations make faster, insight-driven decisions, optimise operational costs, and foster a more data-centric culture across teams.
To illustrate, let’s take a look at how FreshBytes could benefit from adopting lakehouse-powered insights:
Customer Journey Analysis
By linking online behaviour and interaction data with transaction history, FreshBytes could trace the complete customer journey—from product browsing to cart addition to final checkout. This would help identify specific touchpoints where customers were dropping off, such as during the payment stage or after viewing shipping costs.
Segmentation and Personalisation
Leveraging customer segmentation models, FreshBytes could have grouped customers based on behaviour, purchase history, or demographics. They might have discovered that certain segments—like new visitors or mobile users—were more prone to abandon their carts. This would allow for targeted interventions, such as personalised recommendations or tailored discount offers.
Performance Improvements
By leveraging real-time and historical data, FreshBytes could dramatically reduce the time needed for analysis. What once took weeks might now be completed in hours, enabling faster, more responsive decision-making. With real-time monitoring, they could also identify customer issues more quickly, reducing response times from days to minutes.
Cost Efficiency
Through efficient data organisation and query optimisation, FreshBytes could realise significant cost savings, even when dealing with large datasets. For example, compute costs could potentially decrease by 40%, enabling more cost-effective data processing without sacrificing performance.
Business Impact
By identifying and removing duplicate customer records, FreshBytes could reduce marketing costs by approximately 15%, streamlining campaigns and targeting. Additionally, personalised recommendations—driven by segmentation insights—could increase average order value, driving higher revenue per customer. Real-time monitoring could also help the support team proactively assist customers, potentially boosting customer satisfaction scores.
Data-Driven Culture
As FreshBytes continues to leverage lakehouse-powered insights, it could foster a more data-driven culture throughout the organisation. With better access to accurate, real-time data, departments might become more inclined to base their decisions on evidence and insights. This could lead to a 300% increase in data queries across the company, as analytics become more deeply integrated into everyday operations.
From Data to Intelligence
The FreshBytes example illustrates the transformative impact of data transformation within a lakehouse architecture. By converting raw, fragmented data into unified, clean, and insight-ready formats organisations can overcome immediate data challenges and build a strong foundation for growth and innovation. If your organisation aims to turn raw data into actionable intelligence, HorizonX can guide you every step of the way. Contact us today to begin your journey to data excellence.
In our next post, we’ll explore the powerful applications of AI and Machine Learning (ML) in advanced analytics.
Transforming Data for Business Insights: Raw form to intelligence
Building on our previous post about scalable data storage, the next essential step for businesses is data transformation—ensuring data is "insight-ready" for effective decision-making. In this post, we’ll explore the role of data transformation in unlocking valuable insights, using FreshBytes as an example to highlight its benefits and impact.
The limitations of raw data for generating insights
Data often starts life off in raw, unstructured formats that may be incomplete, inconsistent, or dispersed across various systems. Without data transformation, companies face challenges in integrating sources, identifying patterns and drawing reliable conclusions.
FreshBytes needed a comprehensive view of the customer journey to understand issues like cart abandonment, supply chain inefficiencies and customer churn. However, fragmented data from website interactions, purchase history, inventory and loyalty programmes undermined confidence in their insights. This disjointed data limited their ability to forecast inventory and revenue, optimise store performance, and improve customer retention. Additionally, challenges in data quality, governance and collection further prevented FreshBytes from gaining actionable insights to drive targeted actions.
The need for reliable insight ready data
Every organisation, regardless of size, location or industry, needs clean, consistent, and insights-ready data to guide decision-making
Consolidating customer, sales and relevant data into a unified dataset, would enable a company like FreshBytes to gain a clearer picture of customer behaviour. This would allow it to identify the factors driving cart abandonment and take data-informed steps to improve satisfaction, retention and conversion rates.
Leveraging Data Transformation for Business Value
Data transformation is not a one-time task; it demands an ongoing, robust solution capable of handling complex datasets at scale.
Example: FreshBytes Lakehouse for insights
Data Integration
The lakehouse solution would unify data from multiple sources, including daily customer transaction data (500 GB compressed), customer support logs (500 GB daily), and customer records (500 GB daily). Additionally, three years of historical data (approximately 6 PB) could be included to enable a comprehensive analysis of customer behaviour and trends over time.
Data Processing
Using Apache Spark on the lakehouse architecture, raw data would be processed and transformed into analysis-ready datasets. This would involve performing complex joins and aggregations across the entire dataset to eliminate data silos and resolve the processing limitations that previously hindered complete analysis.
Advanced Analytics
Transformed data enables advanced data analytics, supporting predictive insights like inventory demand forecasting, customer behaviour prediction and forecasting resource utilisation. In addition, it offers operational intelligence which would include, real-time monitoring, automated anomaly detection, and dynamic resource allocation—driving proactive decisions and operational efficiency at FreshBytes.
Real-Time Insights
Streaming analytics would enable real-time monitoring of customer behaviour, allowing for immediate actions and adjustments. Automated alerts would notify teams of data inconsistencies, ensuring timely issue resolution and maintaining data accuracy and reliability.
The success behind lakehouse-powered insights
By consolidating real-time and historical data in a single platform, lakehouse solutions can help organisations make faster, insight-driven decisions, optimise operational costs, and foster a more data-centric culture across teams.
To illustrate, let’s take a look at how FreshBytes could benefit from adopting lakehouse-powered insights:
Customer Journey Analysis
By linking online behaviour and interaction data with transaction history, FreshBytes could trace the complete customer journey—from product browsing to cart addition to final checkout. This would help identify specific touchpoints where customers were dropping off, such as during the payment stage or after viewing shipping costs.
Segmentation and Personalisation
Leveraging customer segmentation models, FreshBytes could have grouped customers based on behaviour, purchase history, or demographics. They might have discovered that certain segments—like new visitors or mobile users—were more prone to abandon their carts. This would allow for targeted interventions, such as personalised recommendations or tailored discount offers.
Performance Improvements
By leveraging real-time and historical data, FreshBytes could dramatically reduce the time needed for analysis. What once took weeks might now be completed in hours, enabling faster, more responsive decision-making. With real-time monitoring, they could also identify customer issues more quickly, reducing response times from days to minutes.
Cost Efficiency
Through efficient data organisation and query optimisation, FreshBytes could realise significant cost savings, even when dealing with large datasets. For example, compute costs could potentially decrease by 40%, enabling more cost-effective data processing without sacrificing performance.
Business Impact
By identifying and removing duplicate customer records, FreshBytes could reduce marketing costs by approximately 15%, streamlining campaigns and targeting. Additionally, personalised recommendations—driven by segmentation insights—could increase average order value, driving higher revenue per customer. Real-time monitoring could also help the support team proactively assist customers, potentially boosting customer satisfaction scores.
Data-Driven Culture
As FreshBytes continues to leverage lakehouse-powered insights, it could foster a more data-driven culture throughout the organisation. With better access to accurate, real-time data, departments might become more inclined to base their decisions on evidence and insights. This could lead to a 300% increase in data queries across the company, as analytics become more deeply integrated into everyday operations.
From Data to Intelligence
The FreshBytes example illustrates the transformative impact of data transformation within a lakehouse architecture. By converting raw, fragmented data into unified, clean, and insight-ready formats organisations can overcome immediate data challenges and build a strong foundation for growth and innovation. If your organisation aims to turn raw data into actionable intelligence, HorizonX can guide you every step of the way. Contact us today to begin your journey to data excellence.
In our next post, we’ll explore the powerful applications of AI and Machine Learning (ML) in advanced analytics.

Transforming Data for Business Insights: Raw form to intelligence
Building on our previous post about scalable data storage, the next essential step for businesses is data transformation—ensuring data is "insight-ready" for effective decision-making. In this post, we’ll explore the role of data transformation in unlocking valuable insights, using FreshBytes as an example to highlight its benefits and impact.
The limitations of raw data for generating insights
Data often starts life off in raw, unstructured formats that may be incomplete, inconsistent, or dispersed across various systems. Without data transformation, companies face challenges in integrating sources, identifying patterns and drawing reliable conclusions.
FreshBytes needed a comprehensive view of the customer journey to understand issues like cart abandonment, supply chain inefficiencies and customer churn. However, fragmented data from website interactions, purchase history, inventory and loyalty programmes undermined confidence in their insights. This disjointed data limited their ability to forecast inventory and revenue, optimise store performance, and improve customer retention. Additionally, challenges in data quality, governance and collection further prevented FreshBytes from gaining actionable insights to drive targeted actions.
The need for reliable insight ready data
Every organisation, regardless of size, location or industry, needs clean, consistent, and insights-ready data to guide decision-making
Consolidating customer, sales and relevant data into a unified dataset, would enable a company like FreshBytes to gain a clearer picture of customer behaviour. This would allow it to identify the factors driving cart abandonment and take data-informed steps to improve satisfaction, retention and conversion rates.
Leveraging Data Transformation for Business Value
Data transformation is not a one-time task; it demands an ongoing, robust solution capable of handling complex datasets at scale.
Example: FreshBytes Lakehouse for insights
Data Integration
The lakehouse solution would unify data from multiple sources, including daily customer transaction data (500 GB compressed), customer support logs (500 GB daily), and customer records (500 GB daily). Additionally, three years of historical data (approximately 6 PB) could be included to enable a comprehensive analysis of customer behaviour and trends over time.
Data Processing
Using Apache Spark on the lakehouse architecture, raw data would be processed and transformed into analysis-ready datasets. This would involve performing complex joins and aggregations across the entire dataset to eliminate data silos and resolve the processing limitations that previously hindered complete analysis.
Advanced Analytics
Transformed data enables advanced data analytics, supporting predictive insights like inventory demand forecasting, customer behaviour prediction and forecasting resource utilisation. In addition, it offers operational intelligence which would include, real-time monitoring, automated anomaly detection, and dynamic resource allocation—driving proactive decisions and operational efficiency at FreshBytes.
Real-Time Insights
Streaming analytics would enable real-time monitoring of customer behaviour, allowing for immediate actions and adjustments. Automated alerts would notify teams of data inconsistencies, ensuring timely issue resolution and maintaining data accuracy and reliability.
The success behind lakehouse-powered insights
By consolidating real-time and historical data in a single platform, lakehouse solutions can help organisations make faster, insight-driven decisions, optimise operational costs, and foster a more data-centric culture across teams.
To illustrate, let’s take a look at how FreshBytes could benefit from adopting lakehouse-powered insights:
Customer Journey Analysis
By linking online behaviour and interaction data with transaction history, FreshBytes could trace the complete customer journey—from product browsing to cart addition to final checkout. This would help identify specific touchpoints where customers were dropping off, such as during the payment stage or after viewing shipping costs.
Segmentation and Personalisation
Leveraging customer segmentation models, FreshBytes could have grouped customers based on behaviour, purchase history, or demographics. They might have discovered that certain segments—like new visitors or mobile users—were more prone to abandon their carts. This would allow for targeted interventions, such as personalised recommendations or tailored discount offers.
Performance Improvements
By leveraging real-time and historical data, FreshBytes could dramatically reduce the time needed for analysis. What once took weeks might now be completed in hours, enabling faster, more responsive decision-making. With real-time monitoring, they could also identify customer issues more quickly, reducing response times from days to minutes.
Cost Efficiency
Through efficient data organisation and query optimisation, FreshBytes could realise significant cost savings, even when dealing with large datasets. For example, compute costs could potentially decrease by 40%, enabling more cost-effective data processing without sacrificing performance.
Business Impact
By identifying and removing duplicate customer records, FreshBytes could reduce marketing costs by approximately 15%, streamlining campaigns and targeting. Additionally, personalised recommendations—driven by segmentation insights—could increase average order value, driving higher revenue per customer. Real-time monitoring could also help the support team proactively assist customers, potentially boosting customer satisfaction scores.
Data-Driven Culture
As FreshBytes continues to leverage lakehouse-powered insights, it could foster a more data-driven culture throughout the organisation. With better access to accurate, real-time data, departments might become more inclined to base their decisions on evidence and insights. This could lead to a 300% increase in data queries across the company, as analytics become more deeply integrated into everyday operations.
From Data to Intelligence
The FreshBytes example illustrates the transformative impact of data transformation within a lakehouse architecture. By converting raw, fragmented data into unified, clean, and insight-ready formats organisations can overcome immediate data challenges and build a strong foundation for growth and innovation. If your organisation aims to turn raw data into actionable intelligence, HorizonX can guide you every step of the way. Contact us today to begin your journey to data excellence.
In our next post, we’ll explore the powerful applications of AI and Machine Learning (ML) in advanced analytics.

Download eBook
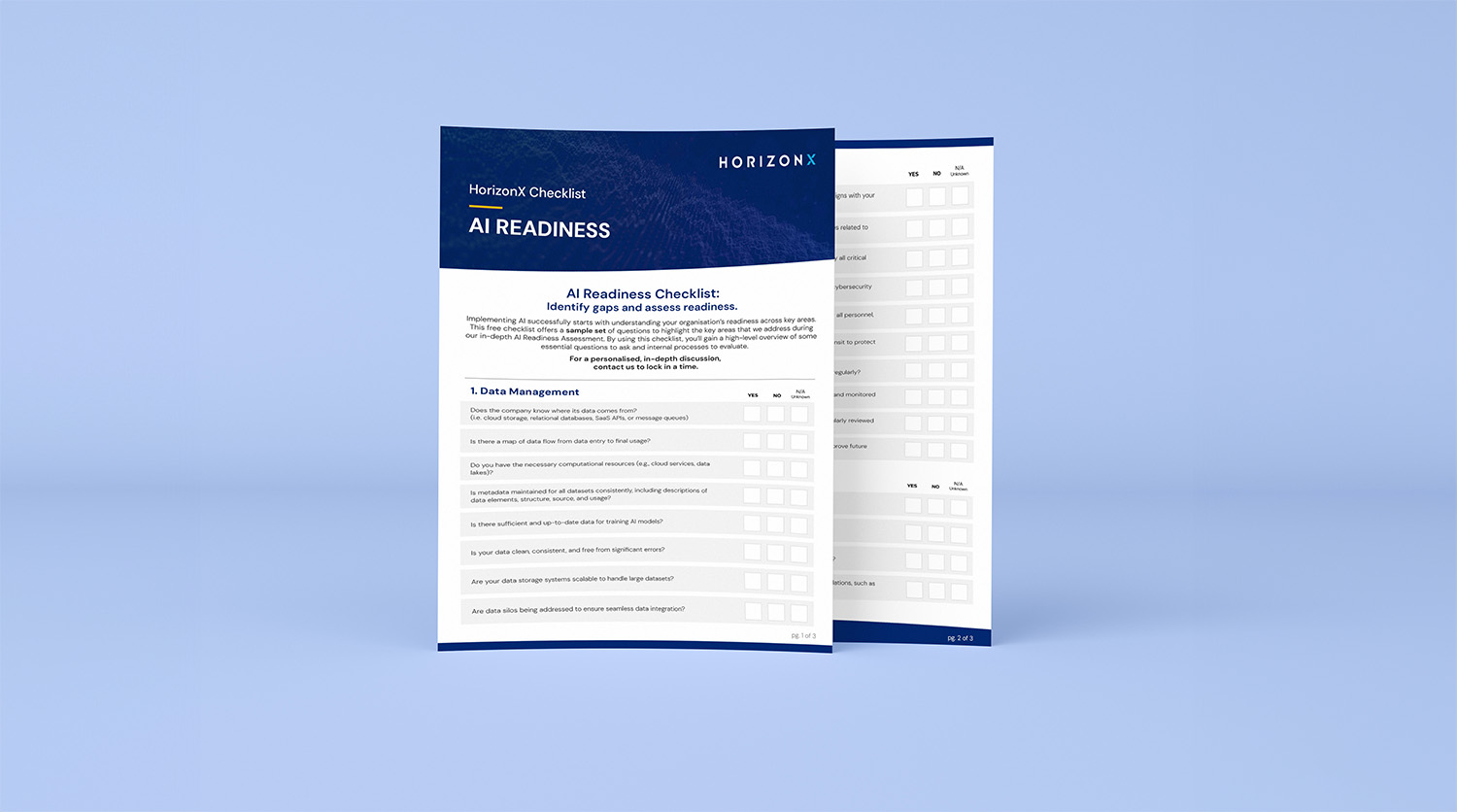
AI Readiness Checklist
Evaluate your organisation’s current state of AI in readiness for AI Adoption. Covering: Data Management, Security, IT Governance and Staff Readiness.
Related Insights
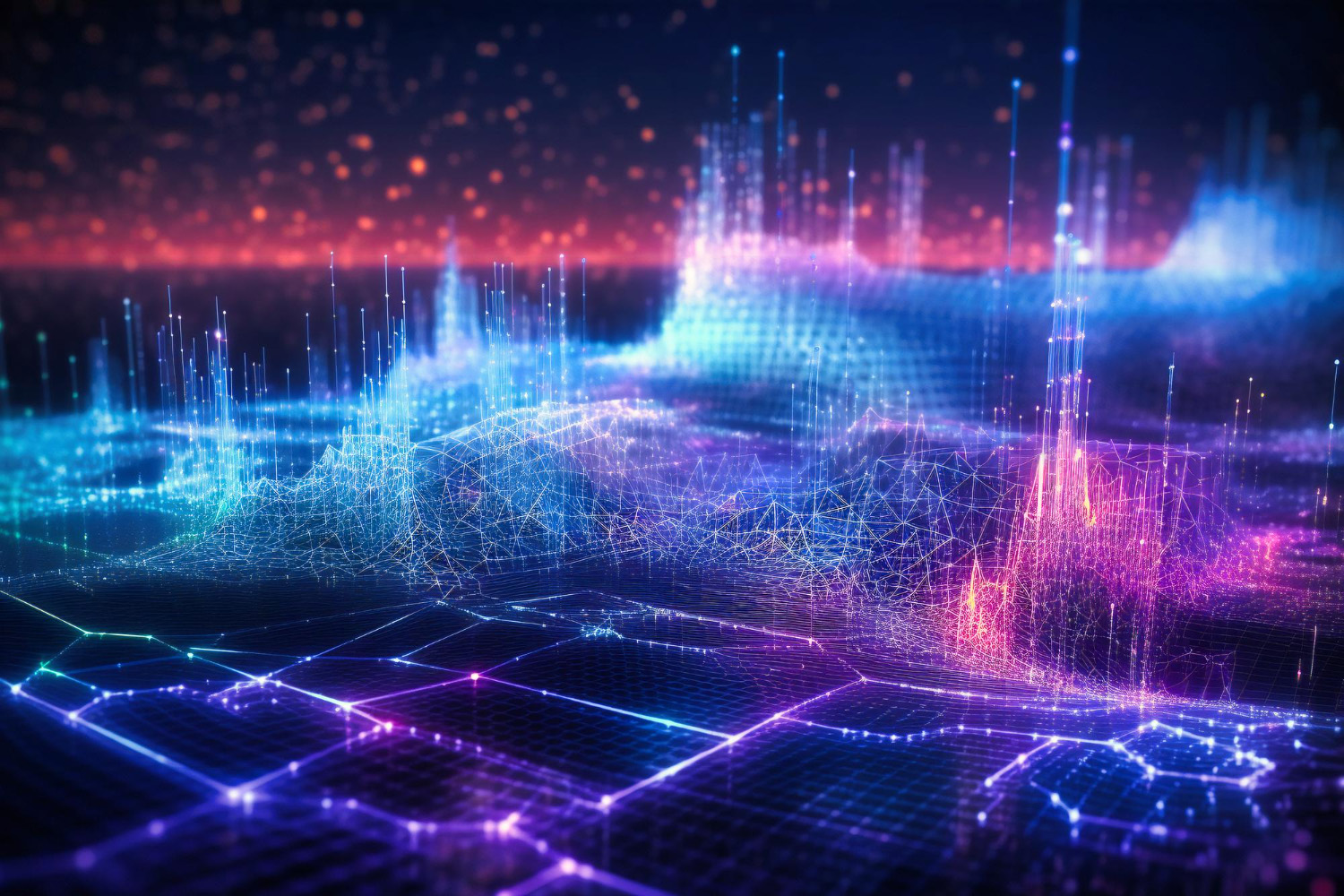
Data Storage: Scaling up for rapid growth.
Explore how Data Lakehouse architecture empowers scalable, cost-effective data storage, as businesses face rapid data growth and rising Cloud costs.
Unlock new opportunities today.
Whether you have a question, a project in mind, or just want to discuss possibilities, we're here to help. Contact us today, and let’s turn your ideas into impactful solutions.