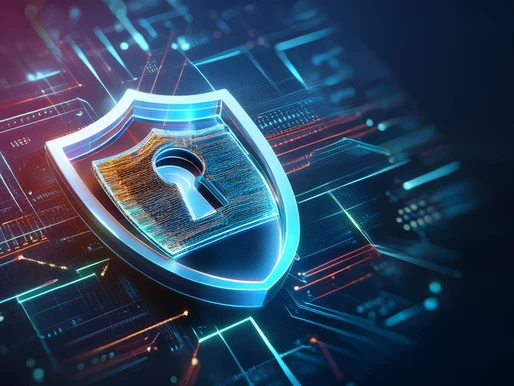
Navigating Data Security in the Gen-AI Era: Balancing Innovation and Protection
As we continue our series on data governance in the era of AI, we now turn our focus to the crucial topic of Data Security. The exponential growth of data means it’s being integrated and shared across more platforms and parties, as well as being used to train AI models. The surge in data highlights the urgent need for robust security measures to protect against financial loss, legal liability, and reputational damage.
AI: A Double-Edged Sword
AI serves as both a powerful ally but also brings significant threats in the realm of data security. While AI enables big data analytics to reach new heights, it also introduces risks when used to process sensitive information. Where humans have historically been able to exercise a high degree of control over data processing, the increased use of AI means this may no longer be the case.
Data Governance for robust Data Security
Data security is a crucial aspect of Data Governance. But how can we achieve scalable data security and governance in an era of data explosion and sophisticated attacks? Here are some key considerations:
Classify Sensitive Data
Technology is making it increasingly easy to collect data from various sources and interactions, often without the user’s knowledge. More of this data will be considered sensitive, and (through AIand ML) it will be correlated with other datasets to draw conclusions - generating even more sensitive data.
Understanding which data requires enhanced protection and identifying at-risk information is essential. Knowing what data you have, its significance, usage, storage location and internal/external consumers (stakeholders, tech-platforms or services) is critical for effective data security practices. Modern metadata management solutions, can assist in classifying and managing data effectively, ensuring a solid foundation for data governance & security practices.
Shared Responsibility Model
As data sharing increases among multiple parties, the importance of a shared responsibility model becomes increasingly important. The model ensures that all parties clearly understand their roles and obligations in data protection, security and regulatory compliance. The most successful companies establish cross-functional teams for their data and analytics governance initiatives.
Access Control
Organisations must implement processes and controls to prevent unauthorised access and misuse of sensitive data. Role-based access control (RBAC) and granular authorisation policies are essential for managing and safeguarding data access. Ensuring the security of data, applications, and models requires a combination of discretionary and role-based access controls. Additionally, anomaly detection can be used to monitor access to applications and data effectively.
Masking and Obfuscation
Advanced data loss prevention (DLP) based features such as row-level security, masking policies, dynamic data masking protect sensitive data while allowing authorised access. Cryptographic techniques safegaurd data in transit and at rest transforming readable data (plaintext) into encoded information (ciphertext). This ensures that even if data is lost, it remains inaccessible to unauthorised parties.
Securing Your AI
While generative AI enhances customer support and data analysis, it also presents security challenges. Training large language models (LLMs) may inadvertently include sensitive, personal, or regulated data. Attackers could manipulate learning data, creating biases, hallucinations, or misinformation, or exploit vulnerabilities in AI models to retrieve data.
Identifying model risks, over-permissive access, and data flow violations throughout the data pipeline is essential.
Identify and Monitor Threats
Implementing robust monitoring and protection systems are essential to stay ahead of security threats. By constantly overseeing data access and usage, organisations can swiftly detect and address potential security risks. This proactive approach not only safeguards sensitive information but also ensures compliance with data protection regulations, reinforcing the overall integrity and reliability of the data governance framework.
AI can help leverage machine learning to analyse data access and usage patterns, identifying unusual activities that may indicate a cyberattack.
In conclusion
A robust data security practice is critical pillar scalable data governance, especially in addressing the demands of the data explosion. As organisations generate vast amounts of data, the complexity of safeguarding against breaches while ensuring compliance with regulatory standards increase. It is essential to review these key considerations to ensure a strong data governance framework.
By establishing a strong data security practice, you can embrace the dual nature of AI, leveraging its strengths while mitigating its risks to safeguard your data assets in this dynamic landscape. In our next blog, we will explore Data Governance through the lens of privacy.
Subscribe for insights
As we continue our series on data governance in the era of AI, we now turn our focus to the crucial topic of Data Security. The exponential growth of data means it’s being integrated and shared across more platforms and parties, as well as being used to train AI models. The surge in data highlights the urgent need for robust security measures to protect against financial loss, legal liability, and reputational damage.
AI: A Double-Edged Sword
AI serves as both a powerful ally but also brings significant threats in the realm of data security. While AI enables big data analytics to reach new heights, it also introduces risks when used to process sensitive information. Where humans have historically been able to exercise a high degree of control over data processing, the increased use of AI means this may no longer be the case.
Data Governance for robust Data Security
Data security is a crucial aspect of Data Governance. But how can we achieve scalable data security and governance in an era of data explosion and sophisticated attacks? Here are some key considerations:
Classify Sensitive Data
Technology is making it increasingly easy to collect data from various sources and interactions, often without the user’s knowledge. More of this data will be considered sensitive, and (through AIand ML) it will be correlated with other datasets to draw conclusions - generating even more sensitive data.
Understanding which data requires enhanced protection and identifying at-risk information is essential. Knowing what data you have, its significance, usage, storage location and internal/external consumers (stakeholders, tech-platforms or services) is critical for effective data security practices. Modern metadata management solutions, can assist in classifying and managing data effectively, ensuring a solid foundation for data governance & security practices.
Shared Responsibility Model
As data sharing increases among multiple parties, the importance of a shared responsibility model becomes increasingly important. The model ensures that all parties clearly understand their roles and obligations in data protection, security and regulatory compliance. The most successful companies establish cross-functional teams for their data and analytics governance initiatives.
Access Control
Organisations must implement processes and controls to prevent unauthorised access and misuse of sensitive data. Role-based access control (RBAC) and granular authorisation policies are essential for managing and safeguarding data access. Ensuring the security of data, applications, and models requires a combination of discretionary and role-based access controls. Additionally, anomaly detection can be used to monitor access to applications and data effectively.
Masking and Obfuscation
Advanced data loss prevention (DLP) based features such as row-level security, masking policies, dynamic data masking protect sensitive data while allowing authorised access. Cryptographic techniques safegaurd data in transit and at rest transforming readable data (plaintext) into encoded information (ciphertext). This ensures that even if data is lost, it remains inaccessible to unauthorised parties.
Securing Your AI
While generative AI enhances customer support and data analysis, it also presents security challenges. Training large language models (LLMs) may inadvertently include sensitive, personal, or regulated data. Attackers could manipulate learning data, creating biases, hallucinations, or misinformation, or exploit vulnerabilities in AI models to retrieve data.
Identifying model risks, over-permissive access, and data flow violations throughout the data pipeline is essential.
Identify and Monitor Threats
Implementing robust monitoring and protection systems are essential to stay ahead of security threats. By constantly overseeing data access and usage, organisations can swiftly detect and address potential security risks. This proactive approach not only safeguards sensitive information but also ensures compliance with data protection regulations, reinforcing the overall integrity and reliability of the data governance framework.
AI can help leverage machine learning to analyse data access and usage patterns, identifying unusual activities that may indicate a cyberattack.
In conclusion
A robust data security practice is critical pillar scalable data governance, especially in addressing the demands of the data explosion. As organisations generate vast amounts of data, the complexity of safeguarding against breaches while ensuring compliance with regulatory standards increase. It is essential to review these key considerations to ensure a strong data governance framework.
By establishing a strong data security practice, you can embrace the dual nature of AI, leveraging its strengths while mitigating its risks to safeguard your data assets in this dynamic landscape. In our next blog, we will explore Data Governance through the lens of privacy.
As we continue our series on data governance in the era of AI, we now turn our focus to the crucial topic of Data Security. The exponential growth of data means it’s being integrated and shared across more platforms and parties, as well as being used to train AI models. The surge in data highlights the urgent need for robust security measures to protect against financial loss, legal liability, and reputational damage.
AI: A Double-Edged Sword
AI serves as both a powerful ally but also brings significant threats in the realm of data security. While AI enables big data analytics to reach new heights, it also introduces risks when used to process sensitive information. Where humans have historically been able to exercise a high degree of control over data processing, the increased use of AI means this may no longer be the case.
Data Governance for robust Data Security
Data security is a crucial aspect of Data Governance. But how can we achieve scalable data security and governance in an era of data explosion and sophisticated attacks? Here are some key considerations:
Classify Sensitive Data
Technology is making it increasingly easy to collect data from various sources and interactions, often without the user’s knowledge. More of this data will be considered sensitive, and (through AIand ML) it will be correlated with other datasets to draw conclusions - generating even more sensitive data.
Understanding which data requires enhanced protection and identifying at-risk information is essential. Knowing what data you have, its significance, usage, storage location and internal/external consumers (stakeholders, tech-platforms or services) is critical for effective data security practices. Modern metadata management solutions, can assist in classifying and managing data effectively, ensuring a solid foundation for data governance & security practices.
Shared Responsibility Model
As data sharing increases among multiple parties, the importance of a shared responsibility model becomes increasingly important. The model ensures that all parties clearly understand their roles and obligations in data protection, security and regulatory compliance. The most successful companies establish cross-functional teams for their data and analytics governance initiatives.
Access Control
Organisations must implement processes and controls to prevent unauthorised access and misuse of sensitive data. Role-based access control (RBAC) and granular authorisation policies are essential for managing and safeguarding data access. Ensuring the security of data, applications, and models requires a combination of discretionary and role-based access controls. Additionally, anomaly detection can be used to monitor access to applications and data effectively.
Masking and Obfuscation
Advanced data loss prevention (DLP) based features such as row-level security, masking policies, dynamic data masking protect sensitive data while allowing authorised access. Cryptographic techniques safegaurd data in transit and at rest transforming readable data (plaintext) into encoded information (ciphertext). This ensures that even if data is lost, it remains inaccessible to unauthorised parties.
Securing Your AI
While generative AI enhances customer support and data analysis, it also presents security challenges. Training large language models (LLMs) may inadvertently include sensitive, personal, or regulated data. Attackers could manipulate learning data, creating biases, hallucinations, or misinformation, or exploit vulnerabilities in AI models to retrieve data.
Identifying model risks, over-permissive access, and data flow violations throughout the data pipeline is essential.
Identify and Monitor Threats
Implementing robust monitoring and protection systems are essential to stay ahead of security threats. By constantly overseeing data access and usage, organisations can swiftly detect and address potential security risks. This proactive approach not only safeguards sensitive information but also ensures compliance with data protection regulations, reinforcing the overall integrity and reliability of the data governance framework.
AI can help leverage machine learning to analyse data access and usage patterns, identifying unusual activities that may indicate a cyberattack.
In conclusion
A robust data security practice is critical pillar scalable data governance, especially in addressing the demands of the data explosion. As organisations generate vast amounts of data, the complexity of safeguarding against breaches while ensuring compliance with regulatory standards increase. It is essential to review these key considerations to ensure a strong data governance framework.
By establishing a strong data security practice, you can embrace the dual nature of AI, leveraging its strengths while mitigating its risks to safeguard your data assets in this dynamic landscape. In our next blog, we will explore Data Governance through the lens of privacy.
Navigating Data Security in the Gen-AI Era: Balancing Innovation and Protection
As we continue our series on data governance in the era of AI, we now turn our focus to the crucial topic of Data Security. The exponential growth of data means it’s being integrated and shared across more platforms and parties, as well as being used to train AI models. The surge in data highlights the urgent need for robust security measures to protect against financial loss, legal liability, and reputational damage.
AI: A Double-Edged Sword
AI serves as both a powerful ally but also brings significant threats in the realm of data security. While AI enables big data analytics to reach new heights, it also introduces risks when used to process sensitive information. Where humans have historically been able to exercise a high degree of control over data processing, the increased use of AI means this may no longer be the case.
Data Governance for robust Data Security
Data security is a crucial aspect of Data Governance. But how can we achieve scalable data security and governance in an era of data explosion and sophisticated attacks? Here are some key considerations:
Classify Sensitive Data
Technology is making it increasingly easy to collect data from various sources and interactions, often without the user’s knowledge. More of this data will be considered sensitive, and (through AIand ML) it will be correlated with other datasets to draw conclusions - generating even more sensitive data.
Understanding which data requires enhanced protection and identifying at-risk information is essential. Knowing what data you have, its significance, usage, storage location and internal/external consumers (stakeholders, tech-platforms or services) is critical for effective data security practices. Modern metadata management solutions, can assist in classifying and managing data effectively, ensuring a solid foundation for data governance & security practices.
Shared Responsibility Model
As data sharing increases among multiple parties, the importance of a shared responsibility model becomes increasingly important. The model ensures that all parties clearly understand their roles and obligations in data protection, security and regulatory compliance. The most successful companies establish cross-functional teams for their data and analytics governance initiatives.
Access Control
Organisations must implement processes and controls to prevent unauthorised access and misuse of sensitive data. Role-based access control (RBAC) and granular authorisation policies are essential for managing and safeguarding data access. Ensuring the security of data, applications, and models requires a combination of discretionary and role-based access controls. Additionally, anomaly detection can be used to monitor access to applications and data effectively.
Masking and Obfuscation
Advanced data loss prevention (DLP) based features such as row-level security, masking policies, dynamic data masking protect sensitive data while allowing authorised access. Cryptographic techniques safegaurd data in transit and at rest transforming readable data (plaintext) into encoded information (ciphertext). This ensures that even if data is lost, it remains inaccessible to unauthorised parties.
Securing Your AI
While generative AI enhances customer support and data analysis, it also presents security challenges. Training large language models (LLMs) may inadvertently include sensitive, personal, or regulated data. Attackers could manipulate learning data, creating biases, hallucinations, or misinformation, or exploit vulnerabilities in AI models to retrieve data.
Identifying model risks, over-permissive access, and data flow violations throughout the data pipeline is essential.
Identify and Monitor Threats
Implementing robust monitoring and protection systems are essential to stay ahead of security threats. By constantly overseeing data access and usage, organisations can swiftly detect and address potential security risks. This proactive approach not only safeguards sensitive information but also ensures compliance with data protection regulations, reinforcing the overall integrity and reliability of the data governance framework.
AI can help leverage machine learning to analyse data access and usage patterns, identifying unusual activities that may indicate a cyberattack.
In conclusion
A robust data security practice is critical pillar scalable data governance, especially in addressing the demands of the data explosion. As organisations generate vast amounts of data, the complexity of safeguarding against breaches while ensuring compliance with regulatory standards increase. It is essential to review these key considerations to ensure a strong data governance framework.
By establishing a strong data security practice, you can embrace the dual nature of AI, leveraging its strengths while mitigating its risks to safeguard your data assets in this dynamic landscape. In our next blog, we will explore Data Governance through the lens of privacy.
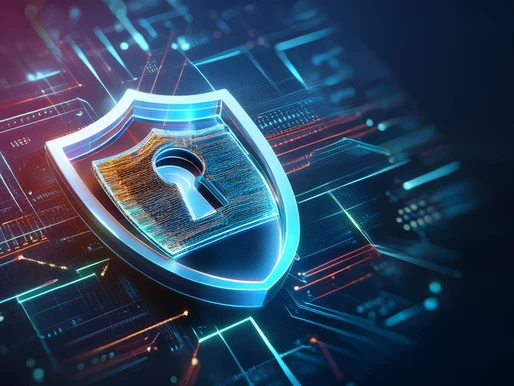
Navigating Data Security in the Gen-AI Era: Balancing Innovation and Protection
As we continue our series on data governance in the era of AI, we now turn our focus to the crucial topic of Data Security. The exponential growth of data means it’s being integrated and shared across more platforms and parties, as well as being used to train AI models. The surge in data highlights the urgent need for robust security measures to protect against financial loss, legal liability, and reputational damage.
AI: A Double-Edged Sword
AI serves as both a powerful ally but also brings significant threats in the realm of data security. While AI enables big data analytics to reach new heights, it also introduces risks when used to process sensitive information. Where humans have historically been able to exercise a high degree of control over data processing, the increased use of AI means this may no longer be the case.
Data Governance for robust Data Security
Data security is a crucial aspect of Data Governance. But how can we achieve scalable data security and governance in an era of data explosion and sophisticated attacks? Here are some key considerations:
Classify Sensitive Data
Technology is making it increasingly easy to collect data from various sources and interactions, often without the user’s knowledge. More of this data will be considered sensitive, and (through AIand ML) it will be correlated with other datasets to draw conclusions - generating even more sensitive data.
Understanding which data requires enhanced protection and identifying at-risk information is essential. Knowing what data you have, its significance, usage, storage location and internal/external consumers (stakeholders, tech-platforms or services) is critical for effective data security practices. Modern metadata management solutions, can assist in classifying and managing data effectively, ensuring a solid foundation for data governance & security practices.
Shared Responsibility Model
As data sharing increases among multiple parties, the importance of a shared responsibility model becomes increasingly important. The model ensures that all parties clearly understand their roles and obligations in data protection, security and regulatory compliance. The most successful companies establish cross-functional teams for their data and analytics governance initiatives.
Access Control
Organisations must implement processes and controls to prevent unauthorised access and misuse of sensitive data. Role-based access control (RBAC) and granular authorisation policies are essential for managing and safeguarding data access. Ensuring the security of data, applications, and models requires a combination of discretionary and role-based access controls. Additionally, anomaly detection can be used to monitor access to applications and data effectively.
Masking and Obfuscation
Advanced data loss prevention (DLP) based features such as row-level security, masking policies, dynamic data masking protect sensitive data while allowing authorised access. Cryptographic techniques safegaurd data in transit and at rest transforming readable data (plaintext) into encoded information (ciphertext). This ensures that even if data is lost, it remains inaccessible to unauthorised parties.
Securing Your AI
While generative AI enhances customer support and data analysis, it also presents security challenges. Training large language models (LLMs) may inadvertently include sensitive, personal, or regulated data. Attackers could manipulate learning data, creating biases, hallucinations, or misinformation, or exploit vulnerabilities in AI models to retrieve data.
Identifying model risks, over-permissive access, and data flow violations throughout the data pipeline is essential.
Identify and Monitor Threats
Implementing robust monitoring and protection systems are essential to stay ahead of security threats. By constantly overseeing data access and usage, organisations can swiftly detect and address potential security risks. This proactive approach not only safeguards sensitive information but also ensures compliance with data protection regulations, reinforcing the overall integrity and reliability of the data governance framework.
AI can help leverage machine learning to analyse data access and usage patterns, identifying unusual activities that may indicate a cyberattack.
In conclusion
A robust data security practice is critical pillar scalable data governance, especially in addressing the demands of the data explosion. As organisations generate vast amounts of data, the complexity of safeguarding against breaches while ensuring compliance with regulatory standards increase. It is essential to review these key considerations to ensure a strong data governance framework.
By establishing a strong data security practice, you can embrace the dual nature of AI, leveraging its strengths while mitigating its risks to safeguard your data assets in this dynamic landscape. In our next blog, we will explore Data Governance through the lens of privacy.
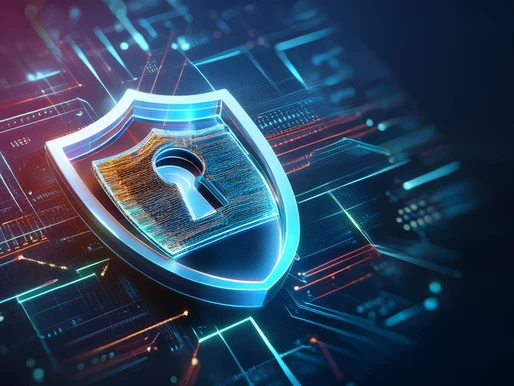
Download eBook
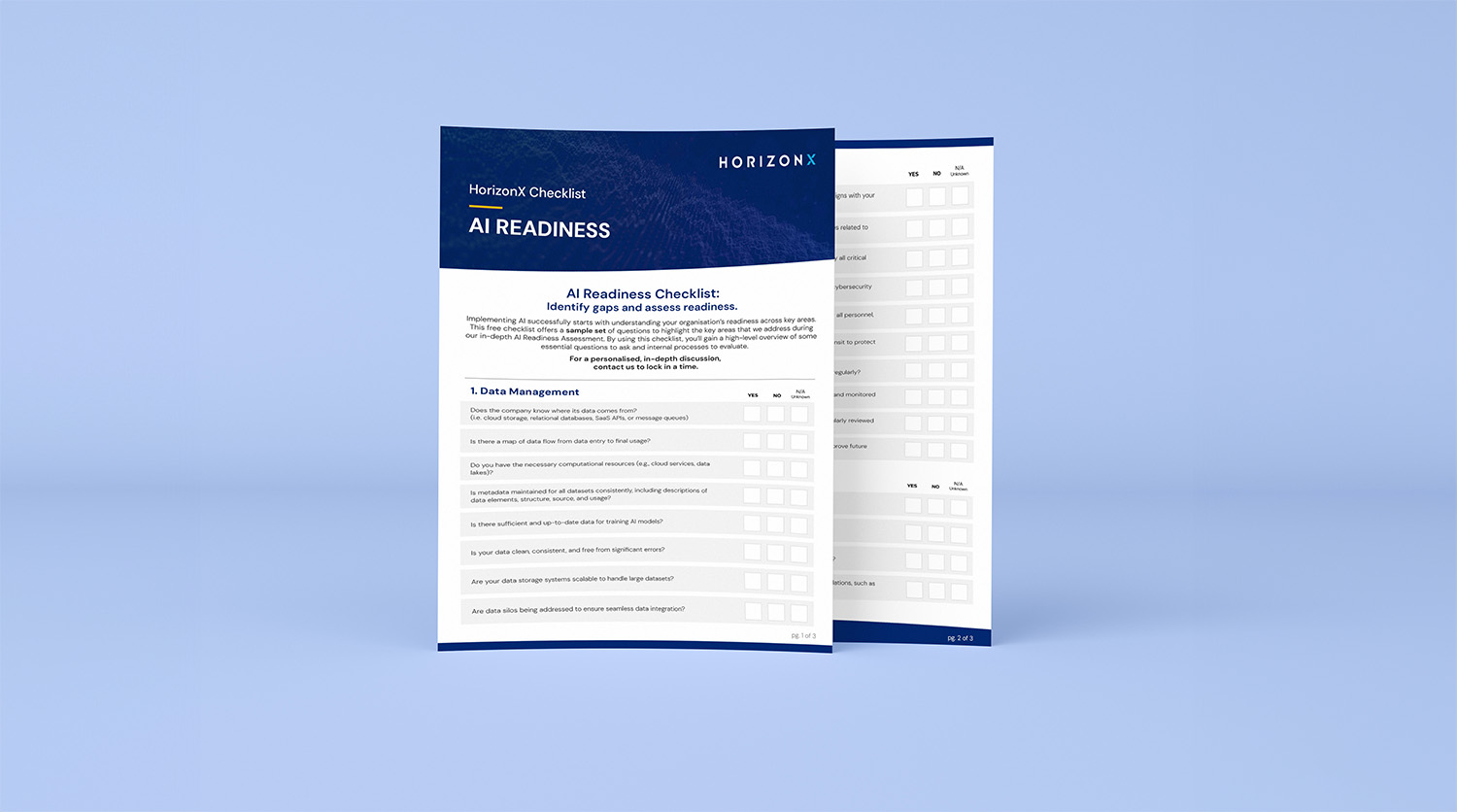
AI Readiness Checklist
Evaluate your organisation’s current state of AI in readiness for AI Adoption. Covering: Data Management, Security, IT Governance and Staff Readiness.
Related Insights
Unlock new opportunities today.
Whether you have a question, a project in mind, or just want to discuss possibilities, we're here to help. Contact us today, and let’s turn your ideas into impactful solutions.