As we expand on our discussion of 'The Data Explosion' and the need for scalable and continuous Data Governance, we now turn our attention to the crucial issue of Data Privacy.

With data privacy laws now enacted in 71% of countries globally, the necessity for robust privacy practices is a given. As both consumer expectations and regulatory demands intensify, organisations must adopt effective privacy measures. These measures not only protect data but also help maintain customer trust, prevent costly fines and preserve the organisation’s reputation.
Here are some key Data Privacy considerations broken down by the data lifecycle phases:
Data Capture
With data often referred to as the “new oil”, there is a strong temptation to and perceived value in collecting as much data as possible. Data privacy best-practice starts with ‘data-minimalisation’ where you collect only the information that is needed for the intended purpose.
Implementing Advanced privacy policies will help ensure that the data collected strikes the right balance between necessity and usability; as more data that is gathered, the greater the legal obligation to ensure compliance and security. Providing a guideline will help team members to efficiently design for and collect targeted data without overstepping that introduces later complications.
Data Storage
Privacy-preserving features must be integrated into storage systems to protect data at rest. These capabilities should be easily accessible, allowing organisations to secure their data without needing deep privacy expertise. Encryption, access controls and automated compliance checks ensure that data remains protected throughout its storage lifecycle.
Data Processing & Usage
When data is processed or analysed, differential privacy plays a crucial role in preserving the utility of the data while protecting individual privacy. By adding statistical noise to data queries, differential privacy allows organisations to conduct analyses and generate insights without revealing sensitive information. This is particularly valuable in AI and machine learning applications, where maintaining data privacy is essential while leveraging the full analytical potential of the data.
Data Sharing/Transfer
During the data sharing phase, data clean rooms provide a secure environment for collaboration. These virtual spaces allow multiple parties to work together on sensitive data without compromising confidentiality, integrity, or security. By controlling access and monitoring interactions within the clean room, organizations can safely share data across departments or with external partners while ensuring that privacy is maintained.
To ensure data protection during sharing, privacy-preserving controls must be enforced. These controls include access restrictions, data masking, and audit trails that monitor how data is used and shared. By implementing these features, organisations can collaborate on data-driven projects without compromising the privacy of the data involved.
Data Archiving
As data moves into the archiving and retention phase, privacy policies such as aggregation and projection continue to play a role. These techniques can be applied to archived data to reduce the risk of exposure while retaining the necessary information for compliance or future analysis. Ensuring that archived data is anonymised or aggregated can further protect privacy during long-term storage.
Data Destruction
When it’s time to dispose of data, privacy capabilities must ensure that data is securely and completely destroyed in a timely manner. Secure deletion methods, such as data wiping or physical destruction, prevent unauthorised recovery of sensitive information. Privacy controls should be enforced to verify that data is not retained longer than necessary, reducing the risk of data breaches.
Data privacy touches every aspect of our lives. Given the ease with which data is now collected, stored and utilised, and the ever increasingly interconnected nature of systems that operate on and share information; it is essential for us, as curators of this information, to protect our customers' data as diligently as we would our own. In our next post we touch on Interoperability as part of our Data Governance series.
As we expand on our discussion of 'The Data Explosion' and the need for scalable and continuous Data Governance, we now turn our attention to the crucial issue of Data Privacy.

With data privacy laws now enacted in 71% of countries globally, the necessity for robust privacy practices is a given. As both consumer expectations and regulatory demands intensify, organisations must adopt effective privacy measures. These measures not only protect data but also help maintain customer trust, prevent costly fines and preserve the organisation’s reputation.
Here are some key Data Privacy considerations broken down by the data lifecycle phases:
Data Capture
With data often referred to as the “new oil”, there is a strong temptation to and perceived value in collecting as much data as possible. Data privacy best-practice starts with ‘data-minimalisation’ where you collect only the information that is needed for the intended purpose.
Implementing Advanced privacy policies will help ensure that the data collected strikes the right balance between necessity and usability; as more data that is gathered, the greater the legal obligation to ensure compliance and security. Providing a guideline will help team members to efficiently design for and collect targeted data without overstepping that introduces later complications.
Data Storage
Privacy-preserving features must be integrated into storage systems to protect data at rest. These capabilities should be easily accessible, allowing organisations to secure their data without needing deep privacy expertise. Encryption, access controls and automated compliance checks ensure that data remains protected throughout its storage lifecycle.
Data Processing & Usage
When data is processed or analysed, differential privacy plays a crucial role in preserving the utility of the data while protecting individual privacy. By adding statistical noise to data queries, differential privacy allows organisations to conduct analyses and generate insights without revealing sensitive information. This is particularly valuable in AI and machine learning applications, where maintaining data privacy is essential while leveraging the full analytical potential of the data.
Data Sharing/Transfer
During the data sharing phase, data clean rooms provide a secure environment for collaboration. These virtual spaces allow multiple parties to work together on sensitive data without compromising confidentiality, integrity, or security. By controlling access and monitoring interactions within the clean room, organizations can safely share data across departments or with external partners while ensuring that privacy is maintained.
To ensure data protection during sharing, privacy-preserving controls must be enforced. These controls include access restrictions, data masking, and audit trails that monitor how data is used and shared. By implementing these features, organisations can collaborate on data-driven projects without compromising the privacy of the data involved.
Data Archiving
As data moves into the archiving and retention phase, privacy policies such as aggregation and projection continue to play a role. These techniques can be applied to archived data to reduce the risk of exposure while retaining the necessary information for compliance or future analysis. Ensuring that archived data is anonymised or aggregated can further protect privacy during long-term storage.
Data Destruction
When it’s time to dispose of data, privacy capabilities must ensure that data is securely and completely destroyed in a timely manner. Secure deletion methods, such as data wiping or physical destruction, prevent unauthorised recovery of sensitive information. Privacy controls should be enforced to verify that data is not retained longer than necessary, reducing the risk of data breaches.
Data privacy touches every aspect of our lives. Given the ease with which data is now collected, stored and utilised, and the ever increasingly interconnected nature of systems that operate on and share information; it is essential for us, as curators of this information, to protect our customers' data as diligently as we would our own. In our next post we touch on Interoperability as part of our Data Governance series.
Complex Data Governance ecosystems and the need for scalable data privacy
As we expand on our discussion of 'The Data Explosion' and the need for scalable and continuous Data Governance, we now turn our attention to the crucial issue of Data Privacy.

With data privacy laws now enacted in 71% of countries globally, the necessity for robust privacy practices is a given. As both consumer expectations and regulatory demands intensify, organisations must adopt effective privacy measures. These measures not only protect data but also help maintain customer trust, prevent costly fines and preserve the organisation’s reputation.
Here are some key Data Privacy considerations broken down by the data lifecycle phases:
Data Capture
With data often referred to as the “new oil”, there is a strong temptation to and perceived value in collecting as much data as possible. Data privacy best-practice starts with ‘data-minimalisation’ where you collect only the information that is needed for the intended purpose.
Implementing Advanced privacy policies will help ensure that the data collected strikes the right balance between necessity and usability; as more data that is gathered, the greater the legal obligation to ensure compliance and security. Providing a guideline will help team members to efficiently design for and collect targeted data without overstepping that introduces later complications.
Data Storage
Privacy-preserving features must be integrated into storage systems to protect data at rest. These capabilities should be easily accessible, allowing organisations to secure their data without needing deep privacy expertise. Encryption, access controls and automated compliance checks ensure that data remains protected throughout its storage lifecycle.
Data Processing & Usage
When data is processed or analysed, differential privacy plays a crucial role in preserving the utility of the data while protecting individual privacy. By adding statistical noise to data queries, differential privacy allows organisations to conduct analyses and generate insights without revealing sensitive information. This is particularly valuable in AI and machine learning applications, where maintaining data privacy is essential while leveraging the full analytical potential of the data.
Data Sharing/Transfer
During the data sharing phase, data clean rooms provide a secure environment for collaboration. These virtual spaces allow multiple parties to work together on sensitive data without compromising confidentiality, integrity, or security. By controlling access and monitoring interactions within the clean room, organizations can safely share data across departments or with external partners while ensuring that privacy is maintained.
To ensure data protection during sharing, privacy-preserving controls must be enforced. These controls include access restrictions, data masking, and audit trails that monitor how data is used and shared. By implementing these features, organisations can collaborate on data-driven projects without compromising the privacy of the data involved.
Data Archiving
As data moves into the archiving and retention phase, privacy policies such as aggregation and projection continue to play a role. These techniques can be applied to archived data to reduce the risk of exposure while retaining the necessary information for compliance or future analysis. Ensuring that archived data is anonymised or aggregated can further protect privacy during long-term storage.
Data Destruction
When it’s time to dispose of data, privacy capabilities must ensure that data is securely and completely destroyed in a timely manner. Secure deletion methods, such as data wiping or physical destruction, prevent unauthorised recovery of sensitive information. Privacy controls should be enforced to verify that data is not retained longer than necessary, reducing the risk of data breaches.
Data privacy touches every aspect of our lives. Given the ease with which data is now collected, stored and utilised, and the ever increasingly interconnected nature of systems that operate on and share information; it is essential for us, as curators of this information, to protect our customers' data as diligently as we would our own. In our next post we touch on Interoperability as part of our Data Governance series.
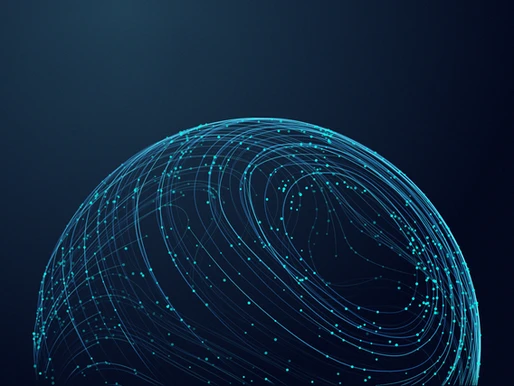
Download Checklist
Complex Data Governance ecosystems and the need for scalable data privacy
As we expand on our discussion of 'The Data Explosion' and the need for scalable and continuous Data Governance, we now turn our attention to the crucial issue of Data Privacy.

With data privacy laws now enacted in 71% of countries globally, the necessity for robust privacy practices is a given. As both consumer expectations and regulatory demands intensify, organisations must adopt effective privacy measures. These measures not only protect data but also help maintain customer trust, prevent costly fines and preserve the organisation’s reputation.
Here are some key Data Privacy considerations broken down by the data lifecycle phases:
Data Capture
With data often referred to as the “new oil”, there is a strong temptation to and perceived value in collecting as much data as possible. Data privacy best-practice starts with ‘data-minimalisation’ where you collect only the information that is needed for the intended purpose.
Implementing Advanced privacy policies will help ensure that the data collected strikes the right balance between necessity and usability; as more data that is gathered, the greater the legal obligation to ensure compliance and security. Providing a guideline will help team members to efficiently design for and collect targeted data without overstepping that introduces later complications.
Data Storage
Privacy-preserving features must be integrated into storage systems to protect data at rest. These capabilities should be easily accessible, allowing organisations to secure their data without needing deep privacy expertise. Encryption, access controls and automated compliance checks ensure that data remains protected throughout its storage lifecycle.
Data Processing & Usage
When data is processed or analysed, differential privacy plays a crucial role in preserving the utility of the data while protecting individual privacy. By adding statistical noise to data queries, differential privacy allows organisations to conduct analyses and generate insights without revealing sensitive information. This is particularly valuable in AI and machine learning applications, where maintaining data privacy is essential while leveraging the full analytical potential of the data.
Data Sharing/Transfer
During the data sharing phase, data clean rooms provide a secure environment for collaboration. These virtual spaces allow multiple parties to work together on sensitive data without compromising confidentiality, integrity, or security. By controlling access and monitoring interactions within the clean room, organizations can safely share data across departments or with external partners while ensuring that privacy is maintained.
To ensure data protection during sharing, privacy-preserving controls must be enforced. These controls include access restrictions, data masking, and audit trails that monitor how data is used and shared. By implementing these features, organisations can collaborate on data-driven projects without compromising the privacy of the data involved.
Data Archiving
As data moves into the archiving and retention phase, privacy policies such as aggregation and projection continue to play a role. These techniques can be applied to archived data to reduce the risk of exposure while retaining the necessary information for compliance or future analysis. Ensuring that archived data is anonymised or aggregated can further protect privacy during long-term storage.
Data Destruction
When it’s time to dispose of data, privacy capabilities must ensure that data is securely and completely destroyed in a timely manner. Secure deletion methods, such as data wiping or physical destruction, prevent unauthorised recovery of sensitive information. Privacy controls should be enforced to verify that data is not retained longer than necessary, reducing the risk of data breaches.
Data privacy touches every aspect of our lives. Given the ease with which data is now collected, stored and utilised, and the ever increasingly interconnected nature of systems that operate on and share information; it is essential for us, as curators of this information, to protect our customers' data as diligently as we would our own. In our next post we touch on Interoperability as part of our Data Governance series.
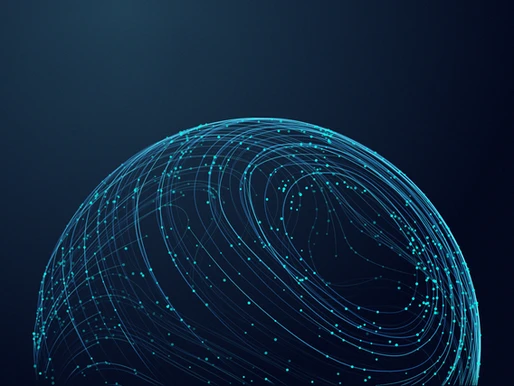
Download eBook

HorizonX Data Governance Checklist
Download our comprehensive Data Governance Checklist: to discover Identify gaps and assessing readiness.
Related Insights
Unlock new opportunities today.
Whether you have a question, a project in mind, or just want to discuss possibilities, we're here to help. Contact us today, and let’s turn your ideas into impactful solutions.